
Maps, the crucial piece of autonomous puzzle, and our investment in Mapillary
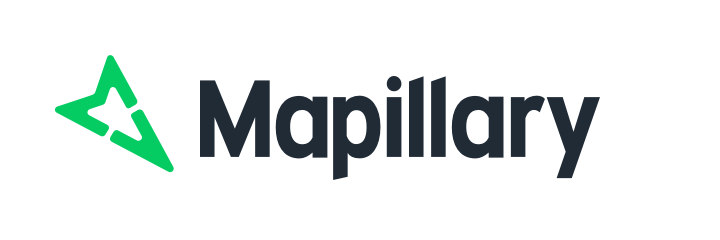
High definition maps play a key role for autonomous driving, yet there are many challenges to tackle. Our investment in Mapillary, an independent provider of street-level imagery and map data, can help address these and make autonomous driving a reality.
Why are maps important?

Maps have played a key role for mobility and trade throughout the history. In ancient Mesopotamia, Babylonians engraved a map on clay tablets to find their way around the holy city of Nippur. Anaximander created a map helping Greek trade ships navigate their way through the Aegean sea. Yet, there is a flurry of recent news on maps... A coalition of carmakers (incl. our parent company), later joined by suppliers all around the world, acquired HERE for several billions dollars. Uber is investing $500M for its global mapping project. Google has acquired Waze for over a billion dollars and has been investing heavily through Waymo and Google Maps. Softbank led a $164M funding round for Mapbox very recently.
Maps, specifically high definition (HD) maps, are at the heart of the autonomous vehicle (AV) ecosystem for a few reasons. First of all, AVs need detailed maps for accurate and precise localization. Today’s GPS technology is far from being perfect, not to mention the issues with the “urban canyon” environment (Yes, this is why your uber shows up a few blocks away). This is especially true under challenging weather conditions, where driving without HD maps is compared to “putting a blind person behind the wheel” by industry analysts. Thanks to HD maps, AVs can match road furnitures and triangulate their position to get centimeter-level accuracy. Another important reason for having detailed maps is sensor redundancy. AVs are equipped with many sophisticated sensors (LiDAR, camera, radar, etc.) and some of these sensors can collect millions of laser points per second resulting in hundreds of GBs of data per hour. Maps can provide foresight, which can lower the workload on sensors and processors. Finally, maps can help AVs see what’s around the corner (e.g. latest accident or construction data) and further down the road. The sensors we’ve mentioned have a limited range- few hundred meters under even the best weather conditions. This means a handful number of seconds if you are driving 70 mph. Maps can integrate real-time traffic information to make the ride safer and more comfortable.
On another note, maps are really interesting from an investing perspective, as map data could be created and consumed by AVs through a common cloud-based platform. This could create a virtuous cycle allowing the solution to get even better and more defensible over time with usage. As highlighted by another investor, “maps have network effects”.
What are the key challenges?
However, creating a detailed map with the precise location of every road furniture, incl. traffic signs, lane markings, among others, and updating it near real time (According to TomTom, about 15% of the roads change every year!) is not a trivial task and there are many key challenges. First, there is a need for huge deployment and we’d need millions of cars on the streets sending their sensor data back to the cloud to create a detailed and up-to-date map. Another challenge is the inconsistency of road furnitures among different locations, which makes the automation of the process even more complicated. The location and structure of the signs change even state by state, e.g. hanging traffic lights are very common in many states, whereas we have poles here, in California. This might sound simple, but think about the scale of this problem if you are a carmaker shipping vehicles all around the world. An additional challenge would be the process of stitching these images/videos from a variety of sensors. Even the angle of the sensors impact the process, not to mention inconsistent capturing quality of a variety of sensors on different vehicles.
On the other hand, today’s traditional map companies are operating in silos, by running their own survey vehicles around the world to collect data and updating their maps accordingly, which is another manual and cumbersome process. This approach is very costly as these survey vehicles are equipped with expensive sensors and they can only cover a small area as this requires a lot of effort. As a result, many different companies cover the same limited areas by incurring huge costs, and no single player ends up having the most accurate data. This results in very slow update cycles, and most of data installed in the vehicles becomes outdated quickly because of this. Additionally, there are cases where certain companies (municipalities, among others) are willing to share map data they’ve collected and can’t find a platform for that.
Our investment in Mapillary
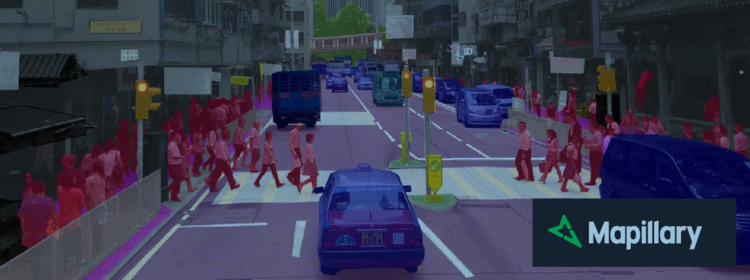
As we were learning more about maps and their challenges, we’ve met Jan Erik Solem, the founder and CEO of Mapillary. Jan Erik has been active in computer vision space since the late 90s. His work on 3D reconstructions for his PhD turned into Polar Rose, a startup providing facial recognition solution running across mobile, cloud and desktop environments. Polar Rose was acquired by Apple in 2010 and the company’s technology helped power some of the latest face detection and recognition APIs and features in Apple products. Following the acquisition, Jan Erik ran a computer vision team at Apple before leaving to start Mapillary. Additionally, he has been a professor at Lund University, and written books about programming and computer vision (one of them still on the top lists after 5+ years).
Jan Erik started Mapillary late 2013 with Yubin Kuang (his former PhD student), Peter Neubauer, and Johan Gyllenspetz. Mapillary has an impressive team, including winners of the Marr Prize, one of the top honors for computer vision researchers. The team publishes their findings and openly shares their data and some of the code on Mapillary’s research website - https://research.mapillary.com/.
Jan Erik describes his vision at a blog post as follows:
“The core idea behind Mapillary is to combine people and organizations with very diverse motives and backgrounds into one solution and one collective photo repository, sharing in the open and helping each other. This means our awesome community, our partner companies and partner organizations, even our customers. That’s right, we’re incentivizing our customers to share their data into the same pool as everyone else, in the open, with an open license...
That we don’t have a mapping or navigation product means we can happily partner with any mapping or navigation company without being in competition. We’re neutral, open, and can work with anyone as long as it benefits our long term vision of visually mapping the planet. This means that photos and data from these partnerships will benefit OpenStreetMap too.”
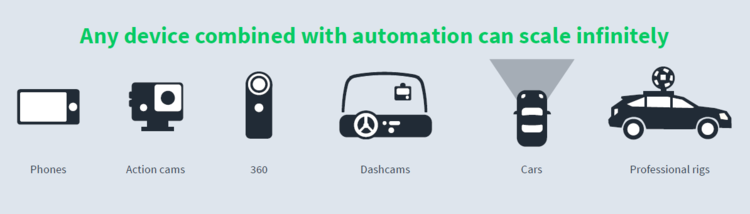
Mapillary is a street-level imagery platform for generating map data at scale through collaboration and computer vision. Mapillary’s technology allows users to upload pictures in a device-agnostic way and generate map data anywhere. Unlike many other players, Mapillary doesn’t need expensive survey vehicles with special gear to achieve quality data. Mapillary has been investing the resources into developing computer vision algorithms, which are capable of of handling data from such a range of different sensors and compensate for any shortcomings of consumer-grade devices. Additionally, it is estimated that there will be 45 billion cameras by 2022, which could fuel the data capturing process further.
Mapillary has a strong network of contributors who want to make the world accessible to everyone by creating a virtual representation of the world. Anybody can join the community and collect street level photos by using simple devices like smartphones or action cameras. This means individuals as well as companies, governments, and NGOs. Recently, facilitating Microsoft’s upload of millions of images quickly, Mapillary’s technology helped with disaster recovery for hurricanes in Florida and Houston. Currently, Mapillary has more than 260M images uploaded, 4M km mapped, 190 countries covered and 22B+ objects recognized with computer vision.
Last May, the company released the Mapillary Vistas Dataset, the world’s largest street-level imagery dataset for teaching machines to see. The dataset includes 25K high resolution images, 100 object categories with global geographic reach under highly variable weather conditions. For autonomous driving, this means that cars will be able to better recognize their surroundings in different street scenes, which in turn helps improve safety. By using this training data and developing new approaches in computer vision research, Mapillary has the best results in semantic segmentation of street scenes, based on two renowned benchmarks.
We believe that there is a strong data network effect for Mapillary’s business. More engagement from community results in more engagement from customers, who contribute images, too. This is further catalyzed by improving computer vision algorithms, developed by Mapillary’s high-caliber research team. As a result, community members and customers extract more value in return and this drives further growth.
Given the challenges we’ve highlighted above, we believe just like Mapillary that there is a need for an independent provider of street-level imagery and map data, which could act as a sharing platform among different players as well. We believe that sharing data is crucial for accurate maps and safer autonomous vehicles as no single player has the necessary deployment in place to ensure this. We are convinced that any company should have access to most accurate map data, and the safety of AV passengers shouldn’t be a differentiator. With the world-class team, passionate community, and unique capabilities, Mapillary is well positioned to address the challenges and help make AV a reality. We couldn’t be more excited to lead this round of investment, and join Atomico, Sequoia and LDV, along with the new investors Navinfo and Samsung to shape the future of mapping!
Baris is an engineer with work experiences in venture capital and top-tier investment banking. At BMW i Ventures, Baris's investing scope encompasses a variety of areas, including industry 4.0, autonomous driving, mobility, AI, digital car/ cloud, customer digital life and energy services. Baris holds a Master of Engineering and Technology Management from Duke University along with an MBA (Dean's Fellow) from UNC Kenan-Flagler Business School, where he led VCIC, the world's largest venture capital competition. Please feel free to reach out on baris@bmwiventures.com.